Table Of Content
A confounding variable is related to both the supposed cause and the supposed effect of the study. It can be difficult to separate the true effect of the independent variable from the effect of the confounding variable. Counterbalancing (randomising or reversing the order of treatments among subjects) is often used in within-subjects designs to ensure that the order of treatment application doesn’t influence the results of the experiment. Second, you may need to choose how finely to vary your independent variable. Sometimes this choice is made for you by your experimental system, but often you will need to decide, and this will affect how much you can infer from your results.
What is the difference between quasi-experimental research and experimental research?
However, because field experiments are not as controlled as laboratory experiments, they may be subject to more sources of error. Laboratory experiments are conducted under controlled conditions, which allows for greater precision and accuracy. However, because laboratory conditions are not always representative of real-world conditions, the results of these experiments may not be generalizable to the population at large. This design involves dividing participants into blocks based on a specific characteristic, such as age or gender, and then randomly assigning participants within each block to one of two or more treatment groups.
Repeated Measures Design
Sometimes randomisation isn’t practical or ethical, so researchers create partially-random or even non-random designs. An experimental design where treatments aren’t randomly assigned is called a quasi-experimental design. A growing trend is to equate study design with only the statistical analysis of the data. In this section, we look at some different ways to design an experiment. The primary distinction we will make is between approaches in which each participant experiences one level of the independent variable and approaches in which each participant experiences all levels of the independent variable. The former are called between-subjects experiments and the latter are called within-subjects experiments.
Statistical experiments, following Charles S. Peirce
It was like someone handed researchers a super-powered magnifying glass, letting them examine multiple studies at the same time to find common trends or results. With Longitudinal Design, instead of measuring something just once, researchers come back again and again, sometimes over many years, to see how things are going. This helps them understand not just what's happening, but why it's happening and how it changes over time. And speaking of success, the factorial design has been a hit since statisticians like Ronald A. Fisher (yep, him again!) expanded on it in the early-to-mid 20th century. It offered a more nuanced way of understanding the world, proving that sometimes, to get the full picture, you've got to juggle more than one ball at a time. Skinner even built boxes—called Skinner Boxes—to test how animals like pigeons and rats learn.
In this type of research, a single group is given a pre-test before a study is conducted and a post-test after the study is conducted. The aim of this one-group pre-test post-test research design is to combine and compare the data collected during these tests. Not all kinds of experimental research can be carried out using simulation as a data collection tool. It is very impractical for a lot of laboratory-based research that involves chemical processes. Only one group of carefully selected subjects are considered in this research, making it a pre-experimental research design example. We will also notice that tests are only carried out at the end of the semester, and not at the beginning.
Design of experiments
Participants in this between-subjects design gave the number 9 a mean rating of 5.13 and the number 221 a mean rating of 3.10. Descriptive (or nonanalytical) studies, as the name suggests, merely try to describe the data on one or more characteristics of a group of individuals. These do not try to answer questions or establish relationships between variables. Examples of descriptive studies include a survey of dietary habits among pregnant women or a case series of patients with an unusual reaction to a drug. Interventional studies are experiments where the researcher actively performs an intervention in some or all members of a group of participants. This intervention could take many forms – for example, administration of a drug or vaccine, performance of a diagnostic or therapeutic procedure, and introduction of an educational tool.
After the question is established, you should state your hypothesis, which should be testable, and explain the objective of the research. Babies do their own rudimentary experiments (such as putting objects in their mouths) to learn about the world around them, while older children and teens do experiments at school to learn more about science. A group, or various groups, are kept under observation after implementing cause and effect factors. You’ll conduct this research to understand whether further investigation is necessary for these particular groups. All rights are reserved, including those for text and data mining, AI training, and similar technologies. For all open access content, the Creative Commons licensing terms apply.
Step 3: Design your experimental treatments
Bike Check: Auckland Cycle Works' "Kind of Like a Rearward Pivot URT" Experimental Prototypes - Pinkbike.com
Bike Check: Auckland Cycle Works' "Kind of Like a Rearward Pivot URT" Experimental Prototypes.
Posted: Wed, 29 Jun 2022 07:00:00 GMT [source]
So, the quasi-experimental approach was like a breath of fresh air for scientists wanting to study complex issues without a laundry list of restrictions. So there you have it—a quick tour through the history of experimental design, from Aristotle's deep thoughts to Fisher's groundbreaking ideas, and all the way to today's computer-powered research. These designs are the recipes that help people from all walks of life find answers to their big questions. Similarly, in research, if you don't have a solid plan, you might get confusing or incorrect results.
Step 5: Measure your dependent variable
Imagine if all the professional-level players ended up on one soccer team and all the beginners on another; that wouldn't be a very informative match! Covariate Adaptive Randomization fixes this by using important traits or characteristics (called "covariates") to guide the randomization process. This design is one of the classics, a staple in research for decades across various fields like psychology, education, and healthcare.
This approach was a lot more reliable than just sitting around and thinking. If you toss ingredients into a bowl without measuring, you'll end up with a mess instead of a tasty dessert. Randomization is important in an experimental research because it ensures unbiased results of the experiment. It also measures the cause-effect relationship on a particular group of interest. By comparing their outcomes in biochemical tests, the researcher can confirm that the changes in the plants were due to the sunlight and not the other variables. Usually, researchers miss out on checking if their hypothesis is logical to be tested.
Nuclear Essentials - World Nuclear Association
Nuclear Essentials.
Posted: Sun, 22 May 2022 14:39:02 GMT [source]
We could then use that information to rank-order participants according to how healthy or unhealthy they are. Next, the two healthiest participants would be randomly assigned to complete different conditions (one would be randomly assigned to the traumatic experiences writing condition and the other to the neutral writing condition). The next two healthiest participants would then be randomly assigned to complete different conditions, and so on until the two least healthy participants. This method would ensure that participants in the traumatic experiences writing condition are matched to participants in the neutral writing condition with respect to health at the beginning of the study.
For example, this research is essential for developing new drugs and medical treatments. Researchers can understand how a new drug works by manipulating dosage and administration variables and identifying potential side effects. A Latin square for an experiment with 6 conditions would by 6 x 6 in dimension, one for an experiment with 8 conditions would be 8 x 8 in dimension, and so on. So while complete counterbalancing of 6 conditions would require 720 orders, a Latin square would only require 6 orders. Surveys can be shared with the respondents both physically and electronically.
This design doesn't just look at one or two things; it looks at several variables simultaneously to see how they interact and affect each other. A high-profile example of Mixed-Methods Design is research on climate change. Scientists use numbers and data to show temperature changes (quantitative), but they also interview people to understand how these changes are affecting communities (qualitative). In a similar way, Crossover Design allows subjects to experience multiple conditions, flipping them around so that everyone gets a turn in each role.
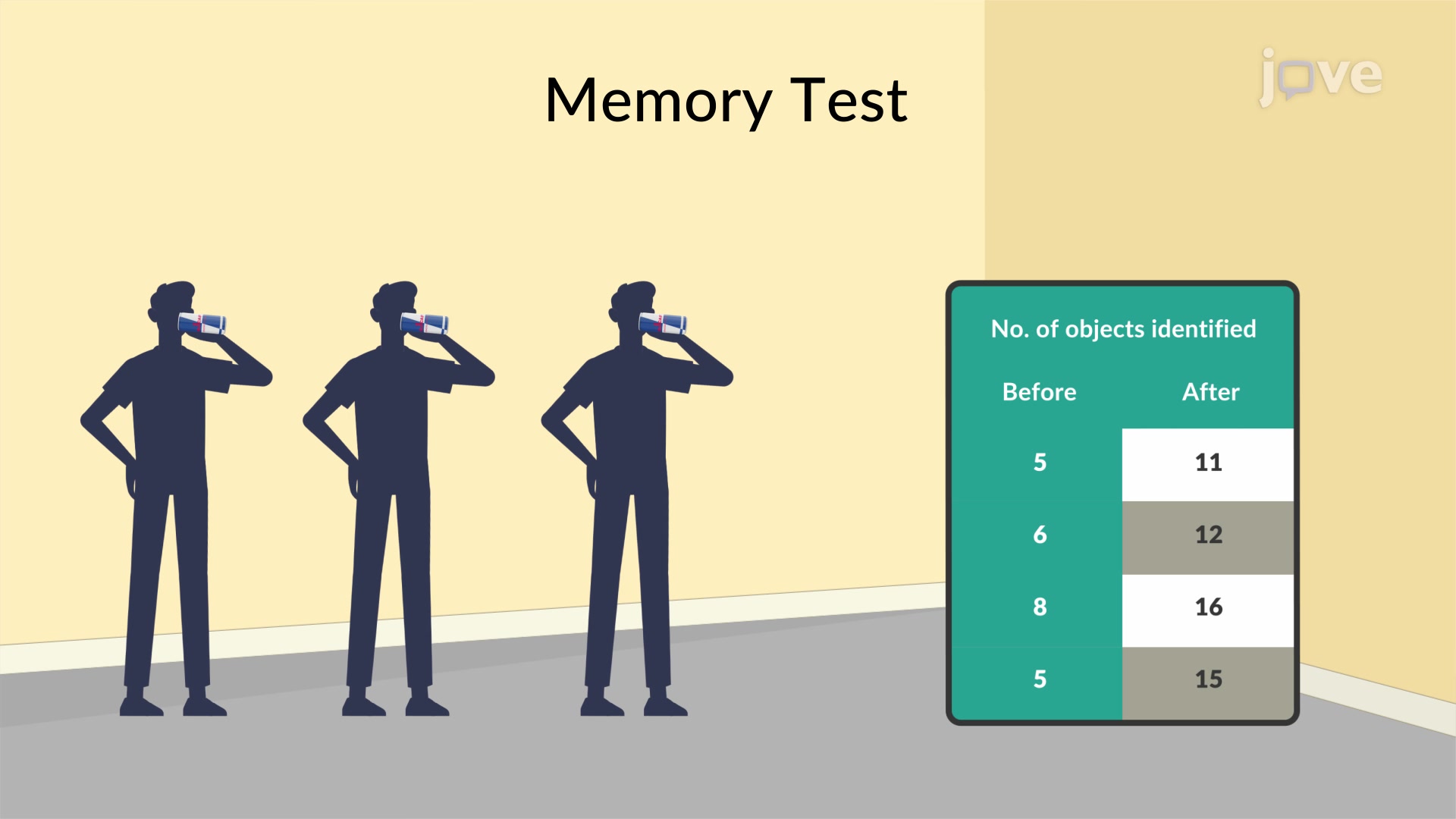
The terms study design, experimental design, and research design are often thought to be synonymous and are sometimes used interchangeably in a single paper. Use the term that is preferred by the style manual of the journal for which you are writing. Study design is the preferred term in the AMA Manual of Style,2 so I will use it here. A proper experimental design serves as a road map to the study methods, helping readers to understand more clearly how the data were obtained and, therefore, assisting them in properly analyzing the results. In a within-subjects experiment, each participant is tested under all conditions. Consider an experiment on the effect of a defendant’s physical attractiveness on judgments of his guilt.
Let's say researchers are testing a new medication for high blood pressure. Participants might have different ages, weights, or pre-existing conditions that could affect the results. The Solomon Four-Group Design is less commonly used than simpler designs but is highly respected for its ability to control for more variables. It's a favorite in educational and psychological research where you really want to dig deep and figure out what's actually causing changes. A well-known example of Crossover Design is in studies that look at the effects of different types of diets—like low-carb vs. low-fat diets. Researchers might have participants follow a low-carb diet for a few weeks, then switch them to a low-fat diet.